Dynamic Sampling Schedule
Machine operators spend a significant amount of time on their daily tasks taking samples to ensure that
the parts produced are in-spec. Typically, the process involves taking a few samples and measuring their dimensions.
Various metrics are used including the raw dimensional values along with process capabilities (CP and CPK). Based
on these metrics, operators may offset a subset of tools to produce in-spec parts. If samples are taken less frequently,
there is a risk of parts going out-of-spec and if samples are taken more frequently, precious operator time is wasted.
In this study, we focused solely on providing optimized sampling schedules for operators relying only on Statistical
Process Control (SPC) data.
The study started by analyzing how every dimension drifts due to the natural physical process as tools wear in.
This needed to account for which tool affects every dimension along with any tool replacements -- the natural
rate of drift various between new and dull tools. Backed by our AI-based analytics, we would then estimate
the number of parts the operator can safely wait for before taking another sample. This is done across all
dimensions. We would then use the minimum value as the one we report to the operator along with the dimension
that will be the first to start going out-of-spec.
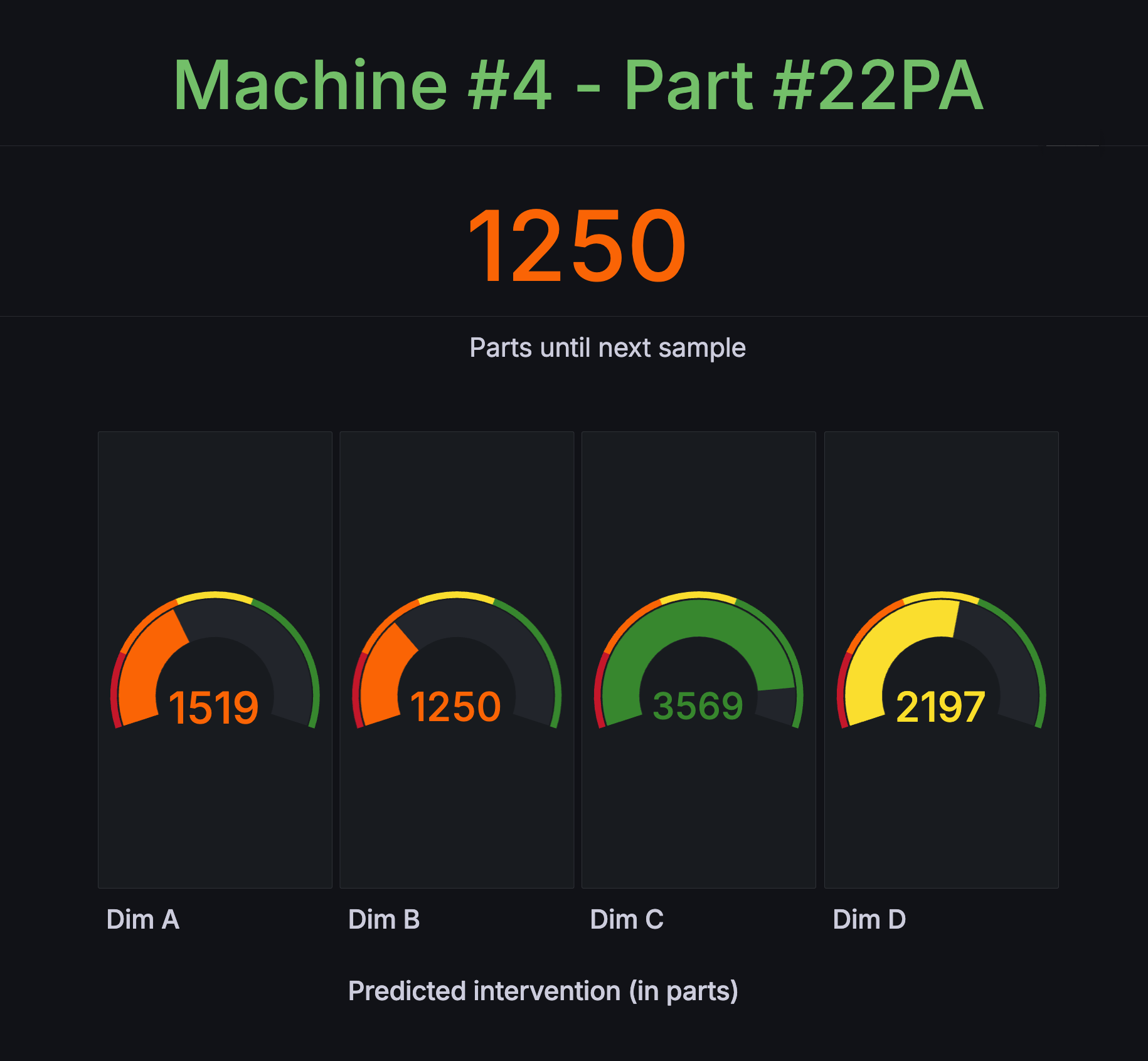
In this study, our analysis showed an increase in the inter-sample time by 56% with only 3% chance that the parts
produced will cross pre-control (a safe region before an operator needs to intervene).
Dynamic sampling schedules allow operators to manage more machines and provide them with a live snapshot of how long each machine can be left without risking the parts going out-of-spec. It also provides the operator with an ordered list of dimensions they need to pay attention to so they can focus on the most critical ones first.